Is there a way to measure the real impact my campaigns have on the revenue?
If you’ve ever asked yourself this question, you probably stumble upon incrementality.
Incrementality represents the increase in sales caused by your ads. It's essential for measuring the true effectiveness of your advertising efforts, providing a clear understanding of how your campaigns, messaging, or specific channels influence revenue. Incrementality testing can boost marketing ROI by 30% says Forrester's report.
In this article, we'll guide you through the process of incrementality testing on Meta. Let’s dive in.
What is incrementality testing and what results it shows?
Incrementality represents the increase in sales caused by your advertising, and incrementality testing is the way to measure these results.
It’s important to understand the crucial difference between what incremental results are, and what they aren’t.
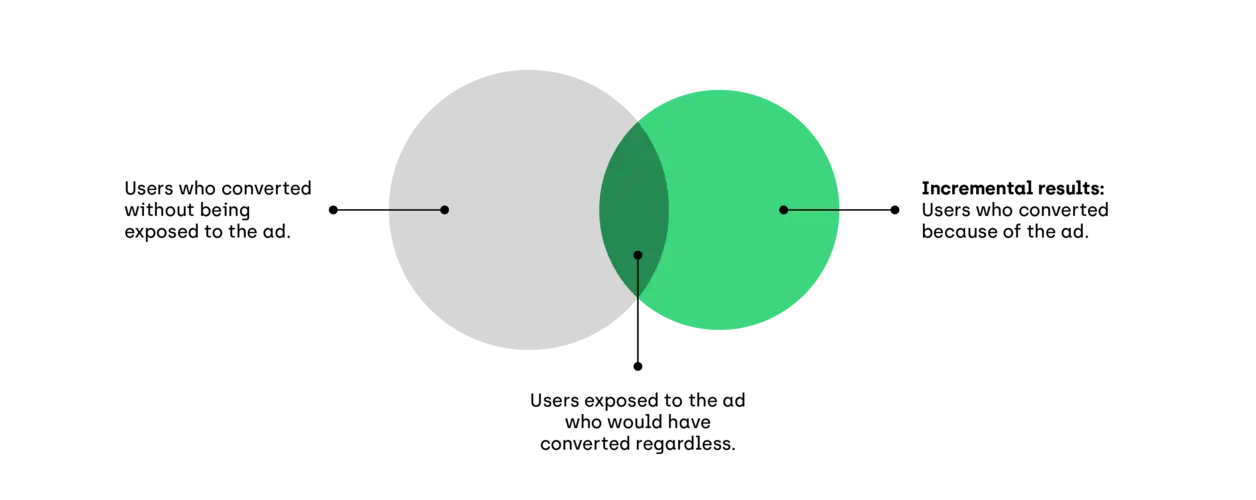
Picture it like this: you’re running a campaign on Facebook, and you measure the campaign’s success through last-touch attribution, meaning that Facebook counts all the conversions that would have happened without being exposed to your ads. These results aren’t incremental.
To put it quite simply:
When your ads help someone to buy a product earlier that’s not incrementality, because that purchase would’ve happened anyway.
The difference between attribution and incrementality measurement
Attribution shows which touchpoints lead to conversions. Since this process is not always that simple, multi-touch attribution has been developed to distribute credit among multiple touchpoints.
On the other hand, incrementality measurement begins by asking whether the conversion would have occurred without that channel. If the answer is yes, it may indicate that you’re wasting your ad spend.
Incrementality testing vs A/B testing: is there a difference?
A/B tests and incrementality tests use similar methodologies but have different objectives.
A/B testing is more versatile, used for measuring and optimizing the performance of a specific campaign, while incrementality testing is used for measuring the effectiveness of the campaign itself and the impact that the campaign has on the desired outcome.
Why should you consider incrementality measurement on Meta?
Incrementality in marketing or advertising can be pivotal in making important business decisions. It provides a clear view of how effectively you are spending your ad budget and shows the impact your ads have on customer behavior.
Incrementality testing reveals the true and often hidden potential of your ads. When making decisions about budget allocation or optimizing your ad spend, incremental results can offer a more precise picture of what's working and what's not.
Relying on the last-click attribution model in such scenarios could be a leap of faith, as it does not precisely measure the impact of your advertising but considers all factors leading to conversion, including organic traffic, Google ads, and other traffic sources.
How does incremental measurement on Meta work?
For incrementality testing, a scientific approach is essential. This involves dividing the audience into two distinct groups, with advertisements shown only to one. On Meta, this stands for Lift measurements.
How does it operate? Initially, Meta randomly splits your target audience into two groups:
- The test group, which is exposed to your ads.
- The control group, which does not see the ads.
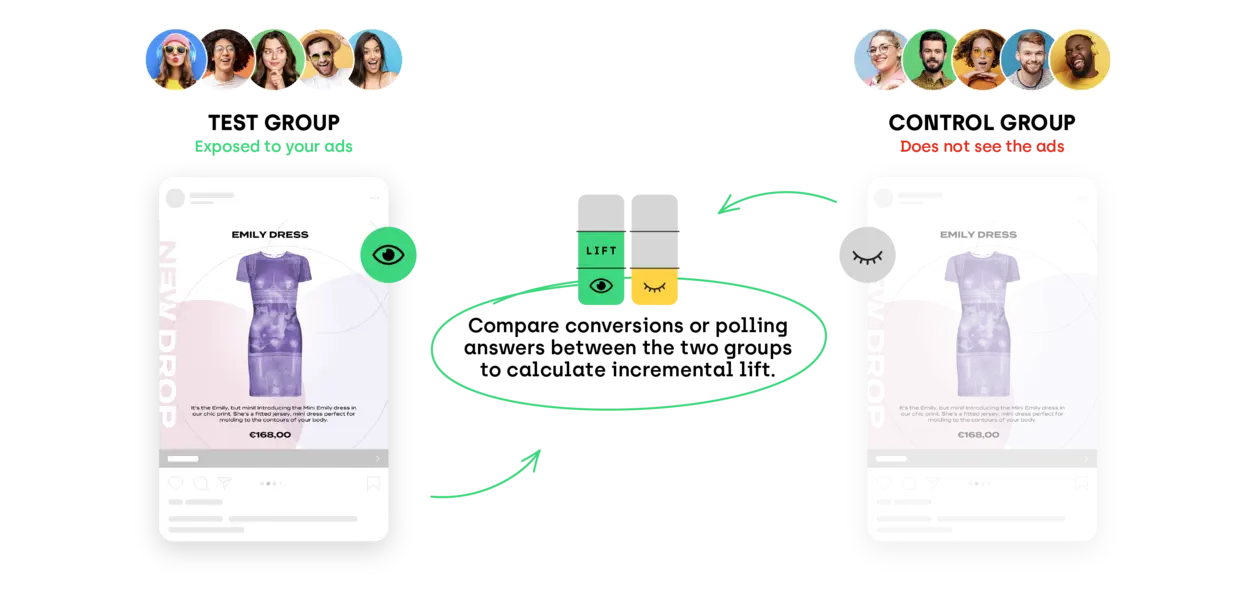
The key focus is measuring performance in each group. The difference in sales between these groups is referred to as incremental lift.
The incremental lift can manifest as positive, neutral, or negative:
- Positive incremental lift indicates superior results in the test group compared to the control group, signifying that the campaign under test is generating revenue and achieving the intended outcome.
- Neutral incremental lift, which is quite self-explanatory, represents identical results for both groups. Such outcomes suggest the need for further testing, possibly involving changes to CTAs, templates, or the overall campaign setup.
- Negative incremental lift, where the control group outperforms the test group, is a rare occurrence. However, it may signal a negative reception of your brand among potential customers. This can happen due to ad fatigue for example.
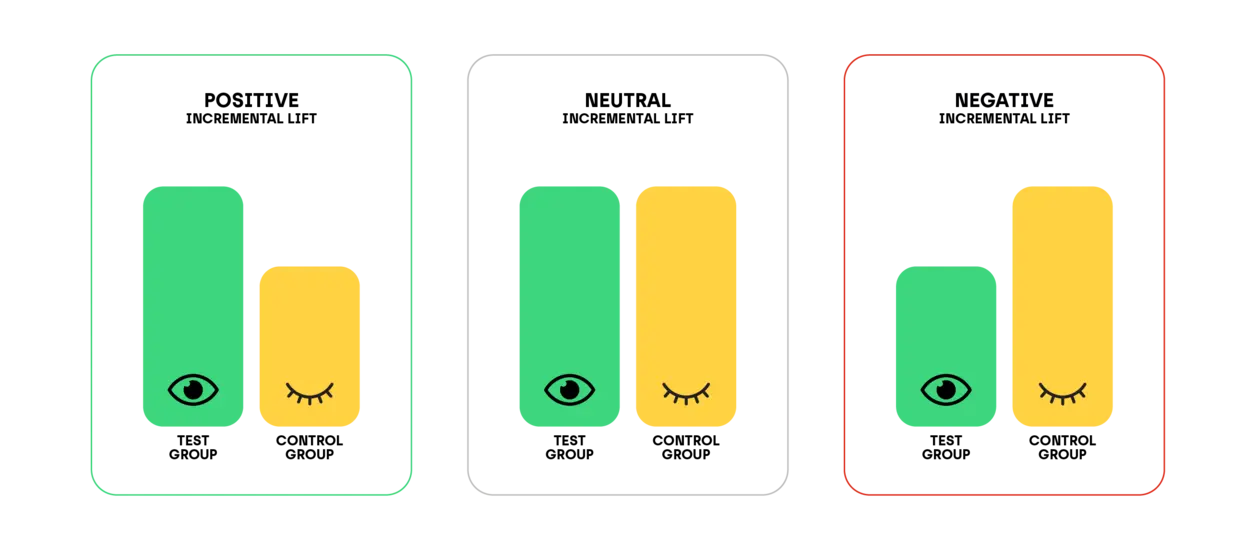
How to do incrementality testing on Meta?
You can test incrementality on Meta in a few ways, and all of them work in the same way: splitting the audience into 2 different groups.
The most recommended one is the Conversion Lift Study.
Conversion Lift Study
Conversion Lift Study works as explained above: Meta randomly splits your target audience into two groups, where the test group sees your ads, and the control group doesn’t. Most tests take 3 to 4 weeks to complete.
While you’ll get pretty accurate results from Meta’s metrics, note that the following will be taken into account:
- Every data outside Meta metrics
- Brand effect
- Lifetime value
- Overall network effect
Conversion Lift Study is pretty much self-service, meaning that you can do most of the work by yourself on Meta. But could you?
Such testing takes time and budget, meaning that all boxes need to be checked and that you have to make sure you did everything right. Because no one wants to be responsible for gambling the team’s budget on the testing that didn’t turn out as expected. Finding the right partner can make this process easier.
The real-life example of Conversion Lift Study with Hunch
Meta's marketing science team conducted a 4-week Conversion Lift Study to measure the lift in ROAS for one of our clients. Using Hunch AI-powered tools, they removed backgrounds from 350,000 product images to create enriched DPAs.
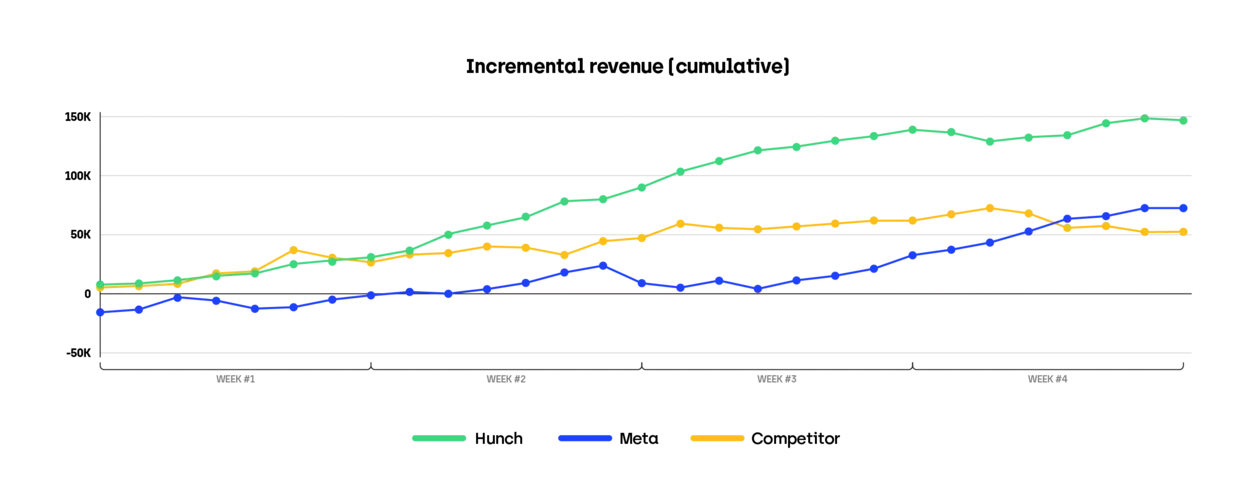
The results of testing showed that Hunch outperformed both competitor overlay and no-overlay templates across all frontiers, driving an incremental ROAS lift of 2.3x. Therefore, partnering with Hunch is estimated to save you 64% in annual costs.
We hope you now have the knowledge to begin experimenting with incremental testing, and we wish you the best of luck. If you have any questions or need our help, reach out!